NumPy: numpy.empty_like() function
numpy.empty_like() function
The numpy.empty_like() function is used to create a new array with the same shape and data type as a given array.
One common use case for numpy.empty_like() is when you want to create a new array with the same shape as an existing array, but with uninitialized values. This can be useful when you need to allocate memory for an array before filling it with data, as it can be faster than creating an array with initialized values.
Syntax:
numpy.empty_like(prototype, dtype=None, order='K', subok=True)
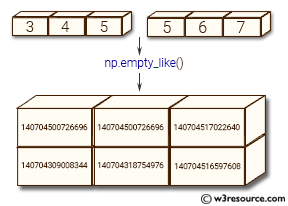
Parameters:
Name | Description | Required / Optional |
---|---|---|
prototype | The shape and data-type of prototype define these same attributes of the returned array. | Required |
dtype | Overrides the data type of the result. | optional |
order | Overrides the memory layout of the result. ‘C’ means Corder, ‘F’ means F-order, ‘A’ means ‘F’ if prototype is Fortran contiguous, ‘C’ otherwise. ‘K’ means match the layout of prototype as closely as possible. New in version 1.6.0. | optional |
subok | If True, then the newly created array will use the sub-class type of 'a', otherwise it will be a base-class array. Defaults to True. | optional |
Return value:
[ndarray] Array of uninitialized (arbitrary) data with the same shape and type as prototype
Example: Create an empty NumPy array using the numpy.empty_like() function
>>> import numpy as np
>>> a = ([1,2,3], [4,5,6])
>>> np.empty_like(a)
array([[139706697202600, 28351168, 139706713266976],
[139706682360248, 139706669155640, 139706713256944]])
>>>
>>> a = np.array([[1., 2., 3.],[4.,5.,6.]])
>>> np.empty_like(a)
array([[ 6.92340539e-310, 9.95630813e-317, 6.92340619e-310],
[ 6.92340619e-310, 1.66406600e-287, 9.67127608e-292]])
>>>
The above code demonstrates the usage of the numpy.empty_like() function to create an empty array with the same shape and data type as the input array.
In the first example, the numpy.empty_like(a) function creates an empty array with the same shape and data type as the input array "a", which is two rows and three columns, and the data type is inferred as an integer. The resulting array is an empty NumPy array of size 2x3 with random values.
In the second example, an input array "a" is defined as a 2D NumPy array with float values. The numpy.empty_like(a) function creates an empty NumPy array with the same shape and data type as the input array "a". The resulting array is a 2x3 empty NumPy array with random float values.
Visual Presentation:
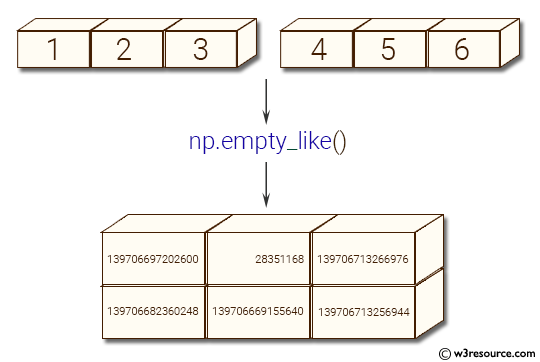
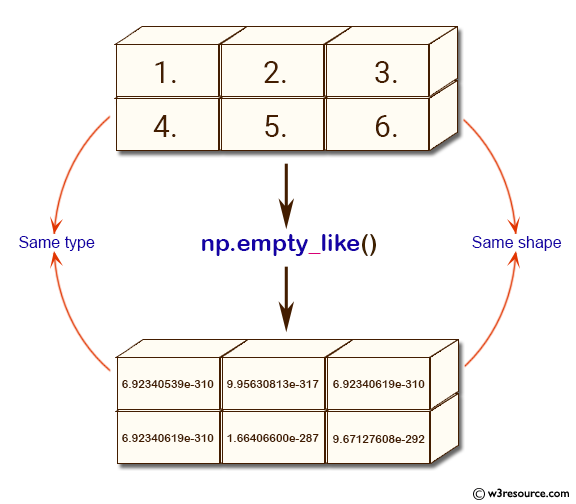
Example: Create an empty NumPy array with the same shape and type as an input array
>>> import numpy as np
>>> a = np.empty_like([2, 2], dtype = int)
>>> print(a)
[139942630210440 139942630210440]
>>>
>>> a = np.empty_like([2, 2], dtype = float)
>>> print(a)
[ 6.93999521e-310 6.93999521e-310]
The above code uses the numpy.empty_like() function to create an empty array with the same shape and data type as the input array. The function takes an array as input and returns a new empty array with the same shape and data type.
In the first example, an empty array is created with the same shape and data type as the Python list [2, 2], with dtype=int. The resulting output shows an array of two elements, with each element being a large integer.
In the second example, an empty array is created with the same shape and data type as the Python list [2, 2], with dtype=float. The resulting output shows an array of two elements, with each element being a very small float value.
Example: Effect of order parameter in np.empty_like() function
>>> import numpy as np
>>> a = np.empty_like([2, 2], dtype = int, order='C')
>>> print(a)
[140209094482824 140209094482824]
>>> a = np.empty_like([2, 2], dtype = int, order='F')
>>> print(a)
[0 0]
>>>
The said code demonstrates the effect of the order parameter in the np.empty_like() function. np.empty_like() returns an empty array with the same shape and data type as the input array.
In the first example, an empty array with the same shape as [2, 2] and integer data type is created with the order parameter set to 'C'. The output shows an array with two elements, both with the same value. This is because the C-contiguous order means the array is filled with elements row-wise, and since the input array is one-dimensional, both elements have the same value.
In the second example, the order parameter is set to 'F', which means the array is filled with elements column-wise. However, the input array is one-dimensional, and thus only two elements are created. As a result, the output array contains two elements with a value of 0.
Example: NumPy.empty_like() using subok parameter (True/False)
>>> import numpy as np
>>> a = np.empty_like([2, 2], dtype = int, order='C', subok=True)
>>> print(a)
[0 0]
>>> a = np.empty_like([2, 2], dtype = int, order='C', subok=False)
>>> print(a)
[0 0]
>>>
In the said code, np.empty_like([2, 2], dtype=int, order='C', subok=True) creates a new array of shape (2,) with data type integer and order C (row-major). Since subok is set to True, it returns an array of the same subclass as the input array. As [2, 2] is a regular Python list and not a NumPy array, the returned array is a base class array of shape (2,) with uninitialized integer values.
Similarly, np.empty_like([2, 2], dtype=int, order='C', subok=False) also creates a new array of shape (2,) with data type integer and order C. However, as subok is set to False, it returns a base class array instead of a subclass of the input array. The resulting array has uninitialized integer values and a shape of (2,).
Frequently asked questions (FAQ) - numpy. empty_like ()
1. What does numpy.empty_like() do?
numpy.empty_like() creates a new array with the same shape and type as a given array, but without initializing its entries.
2. When should I use numpy.empty_like()?
Use numpy.empty_like() when you need an array of the same shape and type as an existing array, but you do not need the elements to be initialized to any particular values.
3. How does numpy.empty_like() handle data type?
numpy.empty_like() will use the data type of the input array by default, but this can be overridden by specifying a different data type.
4. Does numpy.empty_like() initialize the array elements?
No, numpy.empty_like() does not initialize the array elements; the contents are undefined and may contain random data.
5. Can I specify the memory layout for the array created by numpy.empty_like()?
Yes, you can specify the desired memory layout (C-order, F-order, etc.) when creating the array.
6. Is numpy.empty_like() efficient in terms of memory and performance?
Yes, numpy.empty_like() is efficient because it does not initialize array elements, saving time and memory.
7. What are typical use cases for numpy.empty_like()?
Typical use cases include creating arrays for intermediate computations where initialization is not required, improving performance.
8. Are there any similar functions to numpy.empty_like()?
Similar functions include numpy.zeros_like(), which creates an array of the same shape and type but initializes all elements to zero, and numpy.ones_like(), which initializes all elements to one.
Python - NumPy Code Editor: