NumPy: numpy.asarray() function
numpy.asarray() function
The numpy.asarray() function is used to convert a given input to an array.
This is useful when the input may be a list or a tuple, which cannot be used in array-specific operations.
Syntax:
numpy.asarray(a, dtype=None, order=None)
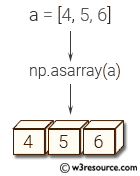
Parameter:
Name | Description | Required / Optional |
---|---|---|
a | Input data, in any form that can be converted to an array. This includes lists, lists of tuples, tuples, tuples of tuples, tuples of lists and ndarrays. | Required |
dtype | By default, the data-type is inferred from the input data. | optional |
order | Whether to use row-major (C-style) or column-major (Fortran-style) memory representation. Defaults to 'C'. | optional |
Return value:
[ ndarray] Array interpretation of a. No copy is performed if the input is already an ndarray with matching dtype and order. If a is a subclass of ndarray, a base class ndarray is returned.
Example: np.asarray() function in NumPy
>>> import numpy as np
>>> a = [2, 3]
>>> np.asarray(a)
array([2, 3])
>>> x = np.array([2, 3])
>>> np.asarray(x) is x
True
In the said code, the variable 'a' is a Python list containing two elements [2, 3]. When np.asarray(a) is executed, it creates a new NumPy array [2, 3]. Similarly, variable 'x' is a NumPy array [2, 3]. When np.asarray(x) is executed, it returns the original array 'x' because it is already a NumPy array.
The 'is' operator is used to check whether two variables refer to the same object in memory. In this case, np.asarray(x) is x returns True because 'x' is already a NumPy array and np.asarray() simply returns the original array without creating a new copy of it.
Visual Presentation:
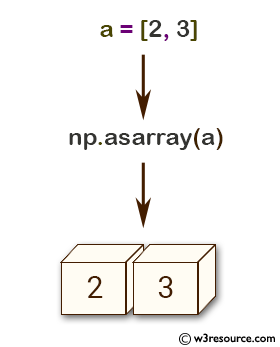
Example: Understanding np.asarray() function in NumPy
>>> import numpy as np
>>> x = np.array([2, 3], dtype=np.float32)
>>> np.asarray(x, dtype=np.float32) is x
True
>>> np.asarray(x, dtype=np.float64) is x
False
In the above code the first line of code creates a NumPy array 'x' [2, 3] and then uses np.asarray() to convert it back into an array. Since 'x' is already an array, the np.asarray() function returns 'x'.
The second line of code converts the array 'x' to a float32 type array and checks whether the returned array is the same as 'x' using the 'is' keyword. Since 'x' is already a float32 type array, np.asarray() returns the same array 'x', so the condition is True.
The third line of code converts the array 'x' to a float64 type array and checks whether the returned array is the same as 'x' using the 'is' keyword. Since the data type of 'x' and the data type passed in np.asarray() is different, np.asarray() creates a new array with float64 data type and returns it, so the condition is False.
Example: Creating and converting structured arrays using NumPy
>>> import numpy as np
>>> issubclass(np.recarray, np.ndarray)
True
>>> a = np.array([(2.0, 3), (3.0, 5)], dtype='f4,i4').view(np.recarray)
>>> np.asarray(a) is a
False
>>> np.asanyarray(a) is a
True
The first line of code checks if the np.recarray class is a subclass of the np.ndarray class, which it is.
The next line of code creates a structured array a with two fields, where the first field is a float32 and the second field is an int32. The view() method is used to convert the array to a record array.
The third line of code checks if np.asarray(a) and a refer to the same object, which is False since np.asarray(a) creates a new NumPy array object.
The final line of code checks if np.asanyarray(a) and a refer to the same object, which is True since np.asanyarray(a) returns a if a is already an array, otherwise it creates a new array object.
Frequently asked questions (FAQ) - numpy. asarray()
1. What is numpy.asarray()?
numpy.asarray() is a function in the NumPy library that converts an input to an ndarray (N-dimensional array). If the input is already an ndarray, it returns the input array without making a copy.
2. When should you use numpy.asarray()?
You should use numpy.asarray() when you need to ensure that your input data is in the form of a NumPy array, but you want to avoid unnecessary copying of data if the input is already a NumPy array.
3. How is numpy.asarray() different from numpy.array()?
numpy.asarray() does not copy the input data if it is already a NumPy array, while numpy.array() always creates a new array, copying the data if necessary.
4. What types of input can numpy.asarray() handle?
numpy.asarray() can handle various input types, including lists, tuples, and other array-like objects. It can also handle scalar inputs by converting them to a 0-dimensional array.
5. Why is numpy.asarray() useful in performance optimization?
numpy.asarray() is useful in performance optimization because it avoids copying data when the input is already a NumPy array, thereby reducing memory usage and improving execution speed.
6. Can numpy.asarray() handle nested sequences?
Yes, numpy.asarray() can handle nested sequences (e.g., lists of lists), converting them into a multi-dimensional NumPy array.
7. What happens if the input to numpy.asarray() is an invalid type?
If the input to numpy.asarray() is an invalid type that cannot be converted to an array, it will raise a ValueError.
8. Does numpy.asarray() allow specifying the data type of the output array?
Yes, numpy.asarray() allows specifying the data type of the output array through an optional argument.
9. Is numpy.asarray() capable of handling memory-mapped files?
Yes, numpy.asarray() can be used with memory-mapped files, facilitating efficient array operations on large datasets stored on disk.
Python - NumPy Code Editor:
Previous: array()
Next: asanyarray()
- Weekly Trends and Language Statistics
- Weekly Trends and Language Statistics